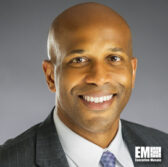
DigitalGlobe has released the results of the second round of its SpaceNet Challenge that aims to accelerate innovation in machine learning technologies.
The company said Monday the SpaceNet Challenge Round 2, hosted by TopCoder, sought automated mapping algorithms that work to extract features of building footprints from overhead imagery.
SpaceNet is a collaborative effort of DigitalGlobe, CosmiQ Works and Nvidia.
Tony Frazier, senior vice president and general manager of DigitalGlobe|Radiant, said competition helps validate the capacity of machine learning and automation to support geospatial analytics applications.
“We are excited to grow the SpaceNet training datasets library for an even broader range of applications and hosting additional public competitions to further machine learning capabilities in the future,” Frazier added.
DigitalGlobe noted that winning algorithms will be available to the open source community via the SpaceNet GitHub repository and the company’s geospatial big data platform GBDX.
The third SpaceNet Challenge will transition from automated building footprint extraction to road network extraction and optimized vehicle routing efforts while the fourth competition will tackle the processing of off-nadir imagery from constellations such as DigitalGlobe’s WorldView Legion.
SpaceNet uses an online repository of satellite imagery, data layers for training algorithms and prize challenges designed to boost machine learning innovation.