“Act locally but learn globally.â€
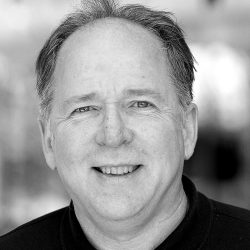
EM: Tell me about your background and how your experience influenced where you are today at MapR.
Ted Dunning: My background is non-traditional in many ways. I started in electrical engineering originally but along the way I took time out of school to help start up a company. This was back before starting up a company in school was the fashion, in the ‘70s. Along the way, I got going on a lot of data-oriented things. What we built back then was an early big data system but it was a lot smaller than what it is now. Since then I’ve done a lot of advanced research particularly around areas where we have lots of data – behavioral analysis either through text or through sequences of symbols on Internet behavior or other kinds of sequences of symbols like genetics. Starting in the ‘90s I began working on a series of start-ups, most fairly successful, mostly exits. Some of the earliest behavioral ad-targeting systems around, early music/video recommendation systems, identity theft detection, fraud detection–the whole sequence of data-oriented start-ups generally based around practical but advanced techniques. Here at MapR we get to work on start-up problems because I get to work with a variety of our customers rather than just working on one company’s problems.
EM: In looking towards the future where do you see MapR having the most significant impact within the federal marketplace?
Ted Dunning: I don’t think the impact is going to be noticeably different in the federal marketplace than it is in a broader commercial marketplace. The applications will be a little bit different. Some of the needs will be fairly strenuous. We have commercial entities asking for as much data capacity as possible to cover their needs. I don’t think there is going to be a huge distinction. The key contribution that MapR is going to be making is has to do with the idea that we provide a solid platform that you can build clusters out of and that allows you to stitch those clusters together into a global data fabric. The ability to have a performing, highly abstracted, easy-to-use data fabric is huge. It is a really big change in how you can do things – the scale and the speed you can work at and the ability to act locally but learn globally. If you have a global data fabric that learning globally becomes much easier. It is an action at a local level, which is relatively easy but moving global models to the local level where action is required can be difficult to do when you have many local locations.
EM: Where has MapR had the greatest success in the federal marketplace?
Ted Dunning: This field that we are talking about is much larger than just Hadoop. Hadoop is just one workload. Hadoop is not a framework. It is a poorly-characterized workload. There are many workloads in the big data world. Hadoop is just one of them. Focusing on Hadoop and doing that well is fine, but you are now working at a very limited part of data at scale, data at speed and data that is geographically distributed. We take a much broader view. We do Hadoop. We do Spark. We do a variety of other workloads on top of our platform–but the platform is the key. Having a platform that handles multiple standard APIs allows you to handle many kinds of workloads. The places that we’ve had impact on such as the IRS are places that have these problems of scale and distribution but also have issues of interfacing with Legacy software. Traditional Hadoop uses HDFS, which only exposes access to files through a very special purpose and idiosyncratic interface, the HDFS-API. It is not suitable for general computation. It segregates a way Hadoop data from ordinary data. That is unfortunate because there are different tools that are appropriate at different times. Most of the tool-building world is not focused around Hadoop. We broke through that by opening up more APIs so you can use non-Hadoop tools with Hadoop techniques or Spark with non-Spark, or SAS with non-SAS. You can run standard databases directly on the platform or you can run all kinds of large data systems. You can do it reliably. So, the key characteristics that help facilitate our successes in the federal space are with customers where losing data really matters, where distributing data really matters and where price/performance really matters.
EM: When you are dealing with a sheer volume of unstructured data, what role does artificial intelligence or machine learning play?
Ted Dunning: I’m a little bit sensitive about the term artificial intelligence. I’ve been through a couple of waves of things being called “artificial intelligence†and then either being discarded or relegated to some other name as they begin to work. Historically, artificial intelligence is the name that people apply to things that don’t work yet. Machine learning, deep learning or cheap learning — these are offshoots of AI that became successful or usable. I am touchy about that term because of its history. You can measure the hype level of different terms by how many brains you see on Google image search when you search for something. When you look at artificial intelligence, everybody has got a picture of some stylized brain — but if you look for machine learning or applied things like that, what you see are mathematics and techniques and images that are being recognized — very concrete stuff. You asked about the interplay of unstructured data and machine learning. In a couple of different ways, you have unstructured or poly-structured or variable structured data which falls under the umbrella of not-so beautifully-structured data. It is becoming dominant. It is becoming much more likely that we will have data that changes its form, is inadequately documented or is variable enough to be called unstructured. That is a fact of the world. As we throw it around much more lightly, we have too much data to fully model and fully control. It is an exercise to do at the limits you can do it. Machine learning is beginning to have a big impact on this.
EM: You just released a book “Streaming Architectureâ€
Ted Dunning: Actually, we released a book Streaming Architecture awhile ago. Then we released another book about geo-distributed data. At the end of September we will be releasing another book called Machine Learning Logistics. In geo-distributed data, the consideration had to do with the fact that we need to measure and act locally, but learn globally. In Machine Learning Logistics, the point of the book is 90 percent of the effort in machine learning is the logistics–the moving of the data to the right place, the capture of training data, the handling of many model versions– all of these logistical get-in-the-way kind of issues are the dominant problems; the noisier parts get in the way much more than the intellectual effort of learning. We provide some practical answers for how to deal with those logistical hurdles.
209631