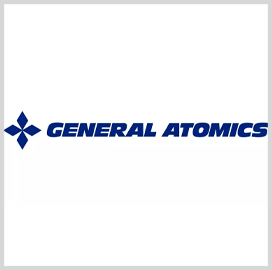
General Atomics researchers have developed a machine learning-based approach to measuring the nuclear fusion uncertainty levels of the DIII-D tokamak device in San Diego.
General Atomics said Monday it used a neural network to allow plasma to operate near its vertical stability threshold to optimize performance while preventing nuclear fusion disruptions.
The researchers leveraged the company’s TokSearch data mining technology to train the neural network using large amounts of data from DIII-D experimental activities.
According to the company, its real-time fusion controls will also support the development of future reactors intended to operate for extended periods of time.
“We’re now starting to be able to use modern machine-learning techniques to augment our physics understanding, and this allows us to control the plasma more effectively,†said Brian Sammuli, one of the researchers for the effort.
“Although DIII-D has applied machine learning to real-time prediction of instabilities for decades, actual real-time control to prevent disruption using these massive data sets is very novel and exciting,†noted David Hill, director of DIII-D.
The tokamak, a device that turns fuel into fusion energy, housed at the DIII-D National Fusion Facility is under the operation of General Atomics on behalf of the Department of Energy’s Office of Science.